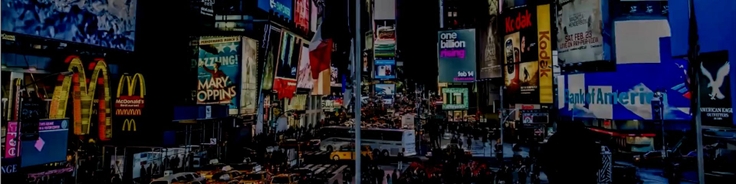
Strata Data Conference in New York brings together thousands of companies across the globe that build their businesses with data. The developers responsible for this revolution need a place to share their experiences on this journey.
This year, Strata Data Conference will offer even more breakout sessions led by data engineers and scientists. This informative mixture of lectures, demonstrations, and guest speakers is geared towards keeping attendees informed on technical content, customer stories, and new launch announcements.
SingleStore is exhibiting in the Sponsor Expo, so stop by our kiosk #2 to view a demo and speak with our subject matter experts.
Here are our top session picks for you to attend at the event.
Geospatial big data analysis at Uber
Zhenxiao Luo (Uber), Wei Yan (Uber)
11:20am–12:00pm, Wednesday, September 27, 2017
Location: 1A 23/24
Uber’s geospatial data is increasing exponentially as the company grows. As a result, its big data systems must also grow in scalability, reliability, and performance to support business decisions, user recommendations, and experiments for geospatial data.
Zhenxiao and Wei will start with an overview of Uber’s big data infrastructure before explaining how Uber models geospatial data and outlining its data ingestion pipeline. They then will discuss geospatial query performance improvement techniques and experiences, focusing on geospatial data processing in big data systems, including Hadoop and Presto.
Zhenxiao and Wei will conclude by sharing Uber’s use cases and roadmap.
Building advanced analytics and deep learning on Apache Spark with BigDL
Yuhao Yang (Intel), Zhichao Li (Intel)
1:15pm–1:55pm, Wednesday, September 27, 2017
Location: 1A 12/14
The rapid development of deep learning in recent years has greatly changed the landscape of data analytics and machine learning and helped empower the success of many applications for artificial intelligence. BigDL, a new distributed deep learning framework on Apache Spark, provides easy and seamlessly integrated big data and deep learning capabilities for users.
Yuhao Yang and Zhichao Li will share real-world examples of end-to-end analytics and deep learning applications, such as speech recognition (e.g., Deep Speech 2), object detection (e.g., Single Shot Multibox Detector), and recommendations, on top of BigDL and Spark, with a particular focus on how the users leveraged the BigDL models, feature transformers, and Spark ML to build complete analytics pipelines.
Yuhao and Zhichao will also explore recent developments in BigDL, including full support for Python APIs (built on top of PySpark), notebook and TensorBoard support, TensorFlow model R/W support, better recurrent and recursive net support, and 3D image convolutions.
When models go rogue: Hard-earned lessons about using machine learning in production
David Talby (Pacific AI)
5:25pm–6:05pm, Wednesday, September 27, 2017
Location: 1A 06/07
Much progress has been made over the past decade on process and tooling for managing large-scale, multi-tier, multicloud apps and APIs, but there is far less common knowledge on best practices for managing machine-learned models (classifiers, forecasters, etc.), especially beyond the modeling, optimization, and deployment process once these models are in production.
Machine learning and data science systems often fail in production in unexpected ways. David Talby shares real-world case studies showing why this happens and explains what you can do about it, covering best practices and lessons learned from a decade of experience building and operating such systems at Fortune 500 companies across several industries.
Topics include:
- Concept drift: Identifying and correcting for changes in the distribution of data in production, causing pretrained models to decline in accuracy
- A/B testing challenges: Recognizing common pitfalls like the primacy and novelty effects and best practices for avoiding them (like A/A testing)
- Offline versus online measurement: Why both are often needed, and best practices for getting them right (refreshing labeled datasets, judgement guidelines, etc.)
Data futures: Exploring the everyday implications of increasing access to our personal data
Daniel Goddemeyer (OFFC NYC), Dominikus Baur (Freelance)
11:20am–12:00pm, Thursday, September 28, 2017
Location: 1E 15/16
Increasing access to our personal data raises profound questions around ownership, ethics, and the resulting sociocultural changes in our everyday lives. Recent legislation that allows the reselling of our personal browser histories without our explicit consent proves the increased need to explore and investigate the consequences that these developments may bring about.
Data Futures, an MFA class in which students observed each other through their own data, explores the social impacts that this informational omnipresence of our personal data may have on our future interactions. In the course, students are guided through a succession of exercises in which they observe each other through their personal data trails to derive assumptions about one another and their class. The changing social dynamics that are exposed by these intimate data exercises showcase how the social behavior of a whole group is affected once personal information becomes accessible. Inspired by this experiential understanding of their data, students then speculate around the future impacts of this knowledge ubiquity by telling visual interaction stories, exemplifying the implications of increasing access to our data.
Daniel Goddemeyer and Dominikus Baur share the findings from Data Futures and demonstrate the results with a live experiment with the audience that showcases some of the effects when personal data becomes accessible.
Executive Briefing: Machine learning—Why you need it, why it’s hard, and what to do about it
Mike Olson (Cloudera)
1:15pm–1:55pm, Thursday, September 28, 2017
Location: 1E 12/13
Companies have been capturing and analyzing data with sophisticated tools for a long time. In recent years, though, two forces have combined to change what’s possible: we can collect and store vastly more data than ever before, and we have powerful new capabilities, like machine learning, to analyze it.
Companies that do this well benefit in many ways. Mike Olson will share examples of real-world machine learning applications, explaining how they matter to business. Mike will also explore a variety of challenges in putting these capabilities into production—the speed with which technology is moving, cloud versus in-data-center consumption, security and regulatory compliance, and skills and agility in getting data and answers into the right hands—and will outline proven ways to meet them.
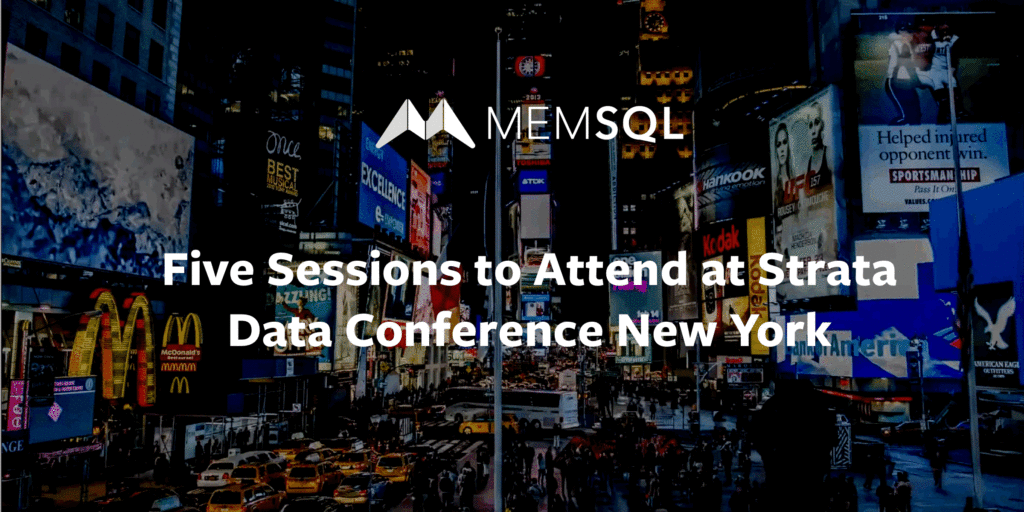