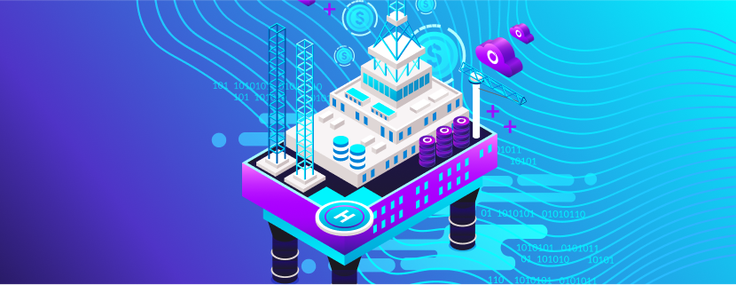
A major oil and gas company works in a volatile market, where IoT data floods in at the rate of gigabytes per second – and where up-to-date financial projections, generated by a .NET application, serve as a major competitive advantage. After considering MongoDB and Oracle Exadata, they replaced two NoSQL data engines at the core of their analytics architecture — Hadoop and ElasticSearch — with SingleStoreDB. After a fast implementation, they now run financial forecasts multiple times a day, with much greater performance, with many fewer servers, and at much lower cost than with competitors. The company now also uses SingleStoreDB for a wide range of financial forecasting applications, land contracts analysis, ERP reporting, and more.
Introduction
Finding, extracting, and delivering oil and gas is one of the most challenging businesses in the world. And it is also, in normal times, one of the most lucrative businesses in the world. But the recent fluctuations in the price of oil, along with a collapse in demand caused by the coronavirus epidemic, have exerted unprecedented pressures on this industry
But oil and gas has always been a tough business. One of the biggest challenges, and opportunities, is financial. Well-run companies use mountains of data to help them manage their reserves of available capital. With good access to capital, when things look good, these companies invest in turning on existing supply, and exploring for new reserves. When times are tough, they reduce production and hold tight until the next opportunity.
Players which are poorly run, or which are too small to compete effectively, tend to miss out on some of the peaks and get caught short in the valleys, driving them to sell out, or go out of business. The assets of the weaker company are then purchased by one of their better-run competitors, which thereby grows larger, and – to the extent that the company is run well – more resilient.
A large oil and gas company has built an extensive Internet of Things (IoT) deployment across its physical plant of wells, drilling rigs, and pipelines. They also use a wide range of external data feeds, and mix this internally generated and market information to make smart decisions. However, a few years ago, their IT infrastructure was not up to the task.
They replaced the databases at the core of their infrastructure with SingleStoreDB. The primary use case for this newly powerful engine is to constantly adjust the company’s financial forecasts. Management is in constant touch with everyone from crews in the field, to lenders and analysts in financial capitals, while scrutinizing roughly 100 major variables at a time. With all of this input, they are constantly creating and stress-testing potential new budgets. All of this forms a company-wide risk exercise, with outsize rewards for success, and severe business consequences for failure.
In this case study, we’ll describe in detail the business problem caused by the previous architecture; what alternative solutions were considered; and how SingleStore helped solve the problem. Now, using SingleStoreDB, the company stands out from competitors, stays ahead of the market and grows, through the best and the worst of times.
This is likely to be of interest to other oil and gas companies; other companies that use IoT in daily operations; companies that need financial analytics and budgeting flexibility, across large and changing data sets; and any company that needs to speed up the flow of information, to meet the current wave of business challenges.
Company Profile
Several years ago, the oil and gas company described here, which is based in Texas, approached SingleStore. This Fortune 500 oil and gas company is publicly held, with tens of billions of dollars in annual revenues and thousands of employees. They actively explore for hydrocarbons – oil and gas – predominantly from shale. Shale sources are notable for being on the expensive side to develop, but are also more easily turned off and on for production than traditional sources of oil and gas. This means they can be actively managed, differently than traditional sources – and elicits the need for constant flows of information to get the best use from the shale resources.
The company uses the data from sensors across the drilling process for real-time well production analysis. They combine this with a wide range of live, always-on, internal and external data feeds to embed themselves in financial and informational data flows. The company can use these massive data flows for a wide range of purposes:
- To quickly repair problems with rigs and other infrastructure
- To predict when maintenance should occur
- To decide when to run which rigs, as prices and customer demand change
- To work with financiers to manage cash and access to capital
The company uses these data flows to reduce non-productive time (NPT) and cut costs – and also to tap capital markets and take advantage of opportunities. But the company’s ability to bring in and process all of this data was severely handicapped by slow and limited data processing operation.
Because of this, decisions that could have saved the company millions of dollars a week – or made the company even more money – went unmade, as the data was not available when and where it was most needed.
Existing resources were weak at every point:
- Ingest capability could not handle the massive flows of internal and external data in a timely fashion.
- Processing capability could not integrate live, streaming data with historical data, costing the company the ability to make decisions using all of the data that it has access to.
- Analytics were slow; in a business where time is money, waiting for answers was intolerable. The company needs to be able to constantly generate and test new budgets, on a region-by-region basis; slow analytics drastically limited flexibility.
- Concurrency for analytics was poor;the company could not support all of its current and desired users at once, to include ad hoc queries, business intelligence (BI) tool queries, and API calls from apps, all hitting the database(s) at the same time. Adding more analytics or BI tool users, or a new app, slowed down results for all users.
The business saw that its weakest point was financial forecasting. If they could integrate all this data to generate solid budgets, and test them against different scenarios so as to best manage risk, they could get ahead of aggressive competition.
After careful study, SingleStoreDB was chosen, to speed ingest, processing, and the delivery of results, with increased concurrency. The new system was scoped, deployed, and brought into production in just seven months. Costs are down; flexibility, manageability, and profitability are up.
The Business Problem
The company is constantly looking for new customers and negotiating with existing ones. Like any public company, they need to meet detailed financial reporting requirements, and to accurately forecast revenues, costs, and profits and losses – per region, and then roll these budgets up for the company as a whole.
None of this is easy. Shale oil company operations are heavily dependent on prices of conventional oil and gas, as production costs must be below the commodity price for a well to be profitable. These costs include drilling and completion costs, delivery costs, operating expenses, taxes, and more. Companies use decline curves to look at the rate of production decline over the life of a well, and work to determine what the estimated ultimate recovery (EUR) will be for a given well. Getting the economics right – not quarter by quarter, but day by day – is a necessity for these companies, given the impact of changing commodity prices.
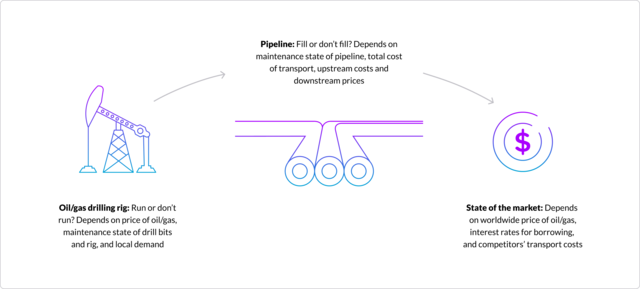
Financial forecasting for an oil and gas company depends on a constantly fluctuating mix of factors.
When the price of oil is high enough, a producing well can be quite lucrative. Today’s digital oilfield uses IoT-enabled devices, sensors, and systems to reduce costs, improve performance, enhance safety, minimize downtime, and drive operational efficiencies. Maintaining extremely complex and expensive equipment, using predictive analytics to do preventive equipment maintenance, and managing the supply chain are all areas that have been improved with IoT.
As a result of the company’s IoT investment, the business is able to manage, and centralize the flow of information from, the myriad of sensors on the drilling rig. Instead of managing each sensor independently, the company can now look holistically at everything from flow rates to temperature and pressure to mud pulse telemetry signals. They are able to profile potential sites extensively and choose which ones to develop. And they can use real-time data to decide when to take rigs online and offline, and when to operate existing wells.
The cost of money – borrowing to fund capital requirements – is another variable. Each region where the company operates wells has its own forecasts and budget. Modeling to support the forecasting and budgeting process for each region is a huge challenge, due to the inherent difficulties of the business, and the sheer number of variables involved (more than 1,000, in many decisions).
Managing and budgeting resources is a critical business requirement. Having the right data infrastructure to power the necessary decision-making processes, and the right people to run the infrastructure, is just as important as having the right equipment and the right people in the field.
With the oil and gas company’s previous infrastructure, they were seeing more than 2x swings in budgetary allocations. That is, the size of opportunities – and the need for funds to pursue them – could easily fluctuate by more than a factor of two during a quarter. The business lost money due to unoptimized decision making, and lost opportunities that they could not see coming up, since the business could not reallocate funds quickly enough. Further, the company could not predict earnings accurately. All of this hurt the company’s financial results.
The Technical Challenge
The company had a wide range of data sources, which overloaded their existing system; growing processing requirements, which were not being met; and ever-increasing analytics demands, both in volume of queries and in number of users. Desired service level agreements (SLAs) for response time were dropping, in the face of stiff competition; SLAs for concurrency were rising, as human analysts, BI tools, financial dashboards, applications, and machine learning models compete for analytics access.
The company used a combination of Hadoop to accumulate incoming data and ElasticSearch to power analytics and the internal opinions of the system capabilities were poor.
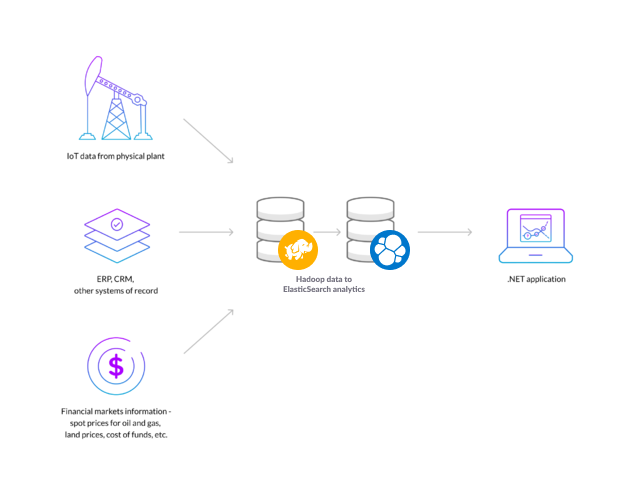
Before: Hadoop and ElasticSearch powered analytics and apps.
ElasticSearch is “a distributed, open source search and analytics engine for all types of data, including textual, numerical, geospatial, structured, and unstructured,” built on Apache Lucene. ElasticSearch is valuable for use with mixed data, but it’s a NoSQL solution, about which we’ve expressed our opinion before. Relational databases that support SQL tend to be much faster for running analytics, per machine, as well as far more compatible. Distributed SQL solutions, such as SingleStoreDB, take this efficiency and scale it smoothly across distributed clusters of generic servers.
Faced with the poor results they were experiencing, the company considered four alternative solutions, in addition to SingleStoreDB. One of the alternatives, Oracle, is a relational database solution; the other three were NoSQL solutions. The alternatives to SingleStoreDB were quickly found wanting:
- Expanded use of Elasticsearch for analytics. Elasticsearch lacks ACID guarantees – basically, the ability to do updates reliably – and true SQL capabilities. Performance is not up to requirements, and scalability also falls short.
- Expanded use of Hadoop processing. Hadoop distribution has the same overall characteristics and concerns as Elasticsearch, as well as ran into serious business problems at the company that sold and supported the software.
- MongoDB. A third NoSQL contender, MongoDB is more widely used for mainstream workloads than the other two. However, Mongo still suffers from a lack of ACID guarantees and true SQL capabilities. Performance falls short.
- Oracle on Exadata. Scaling Oracle is expensive and inefficient. Neither price nor performance of Exadata were appealing to the company.
- SingleStoreDB. SingleStoreDB, the world’s fastest real-time distributed SQL database delivered the price, performance, and SQL support that the company needs, as described below. SingleStoreDB scales much more smoothly, for instance, than Oracle, delivering ten times the performance at one-fourth the cost.
Solving the Problem
The oil and gas company realized they had a big problem, deserving of investment at the company level. So they started by setting out the requirements for a solution:
- CEO-level visibility
- Global availability – more than 300 in initial named users
- Massive data volumes, including bulk ingest at approx 8GB/sec
- Trickle feeds updating millions of rows (very wide tables) per second
- Total compute – 2 clusters, 3200 CPUs, 30TB of Memory
- Interactive analytics – less than 3 seconds to display charts
- Integrate with Oracle, Spark, and Hadoop
- Support for financial application written in .NET
- Fastest possible time to go live (just 7 months, for SingleStoreDB)
SingleStoreDB was considered carefully, and was found to not only solve the core business problem, but several additional problems as well. Here are key technical characteristics of the SingleStoreDB solution:
- Fast time to market. A target was set of seven months, from the start of the deployment effort to deploying the first version of the solutions.
- System performance. Performance requirements were met from day one. Also, unlike other vendors considered, there was no need for outside consulting services to optimize performance and meet the requirement.
- Easy integration. The SingleStoreDB solution integrated with existing systems — Oracle, .NET, and their existing Hadoop infrastructure — out of the box.
- Simplicity. SingleStoreDB was used to replace Elasticsearch and Hadoop for database vendor and database product consolidation, simplicity, and performance.
- Easy scale-out. Growth from 20 systems to 40 systems was accomplished overnight.
- Easy re-use of existing skills. In-house Oracle skills were easily used to manage SingleStoreDB systems.
In contrast to the previous use of NoSQL solutions (Elasticsearch and Hadoop), SingleStoreDB delivered the familiarity of SQL, the ACID capabilities inherent to a relational database management system (RDBMS), and the performance and scalability of a distributed system – an attribute of SingleStoreDB that is generally found in NoSQL solutions, and not generally found in truly SQL-compliant systems, but a key part of what SingleStoreDB delivers.
Price-performance has proven to be excellent. The closest competitor to SingleStoreDB, in terms of capabilities, was Oracle Exadata. But SingleStoreDB consistently excels over Oracle on many attributes, especially price-performance. Many organizations achieve as much as ten times the performance of Oracle at roughly one-fourth the cost.
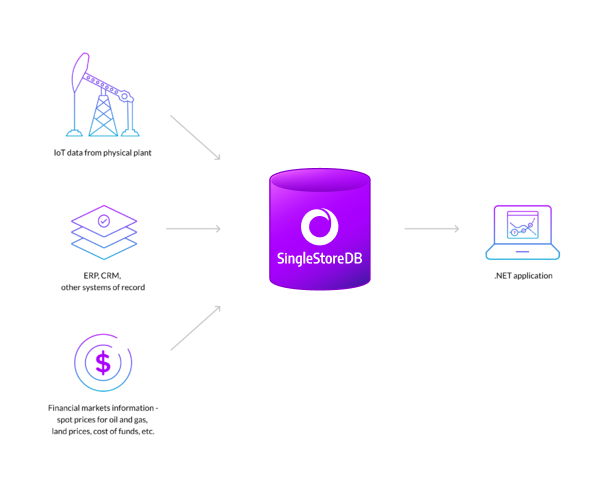
After: SingleStoreDB powers analytics and apps
The business benefits that the company has gained with the move to SingleStoreDB are substantial, and becoming even more significant with time:
- Each department and each region now has the ability to assess current business and oil production, enabling them to forecast budgets from their computers or mobile devices at any time of day.
- With this flexibility, forecasts and budgets are now a major competitive advantage for the company; they can leverage available capital and their physical plant more efficiently than others, and with greater responsiveness to market conditions.
- The volatility of oil and gas prices having only increased in recent years, this flexibility helps separate winners, like this company, from others.
The move to SingleStoreDB has contributed to strong earnings and profitability, as shown in the company’s market capitalization and share price – which consistently rises in good times, and stays ahead of the competition when times are tough.
After the first successful IoT project, this leading oil company has greatly expanded the use of SingleStoreDB across a wide range of applications: additional financial forecasting use cases; land contracts analysis; ERP reporting; and more.
Conclusion
The adoption of SingleStoreDB at this leading oil and gas company led to many breakthroughs; improved performance, a simpler system design, new capabilities, and a new data backbone for a wide range of applications. Today, SingleStoreDB is being considered for ever-broader use within the company.
If you are interested in finding out more about SingleStore’s applicability to your data processing and analytics challenges, try SingleStoreDB for free today or contact SingleStore.