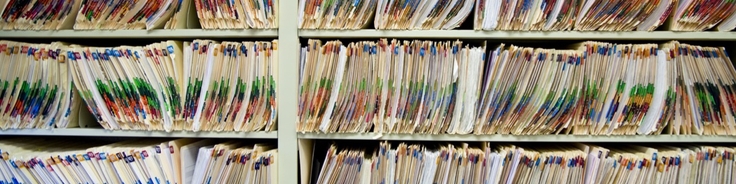
In the Beginning
Years ago, organizations used transactional databases to run analytics. Database administrators struggled to set up and maintain OLAP cubes or tune report queries. Monthly reporting cycles would slow or impact application performance because all the data was in one system. The introduction of custom hardware, appliance-based solutions helped mitigate these issues, and the resulting solutions were transactional databases with column store engines that were fast. Stemming from these changes, several data warehouse solutions sprang up from Oracle, IBM Netezza, Microsoft, SAP, Teradata, and HP Vertica, but these data warehouses were designed for the requirements of 20 years ago. Thus new challenges arose, including:
Ease of use – each environment required specialty services to setup, configure, and tune
Expensive – initial investments were high and needed additional capacity
Scalability – performance was designed on single box configurations; the larger the box, the faster the data warehouse
Batch ingestion – inability to store and analyze streaming data in real-time
As new data or user requests landed on the system, database administrators (DBA) had to scale the system up from a hardware perspective. Need more scale? Buy more boxes! DBAs became tired of having to buy new expensive hardware every time their query was slow or every time they had to ingest from a new data source.
An Explosion of Data
The data warehouse appliance was passable back then, but today, new workloads and data growth have put a strain on traditional solutions to the point where many users are seeking rescue from the clutches of incumbent systems. Explosive data growth due to web and mobile application interactions, customer data, machine sensors, video telemetry, and cheap storage means customers are storing “everything,” this has contributed to the additional strain on traditional systems. Real-time application data, now pervasive in digital business, along with new machine and user generated data puts an increasing pressure on ingestion and query performance requirements. Real-world examples include digital personalization required of retailers, customer 360 programs, real-time IoT applications, and real-time logistic applications. To solve for the increased strain, there has been a strategic shift to cloud and distributed systems for agility and cost optimization.
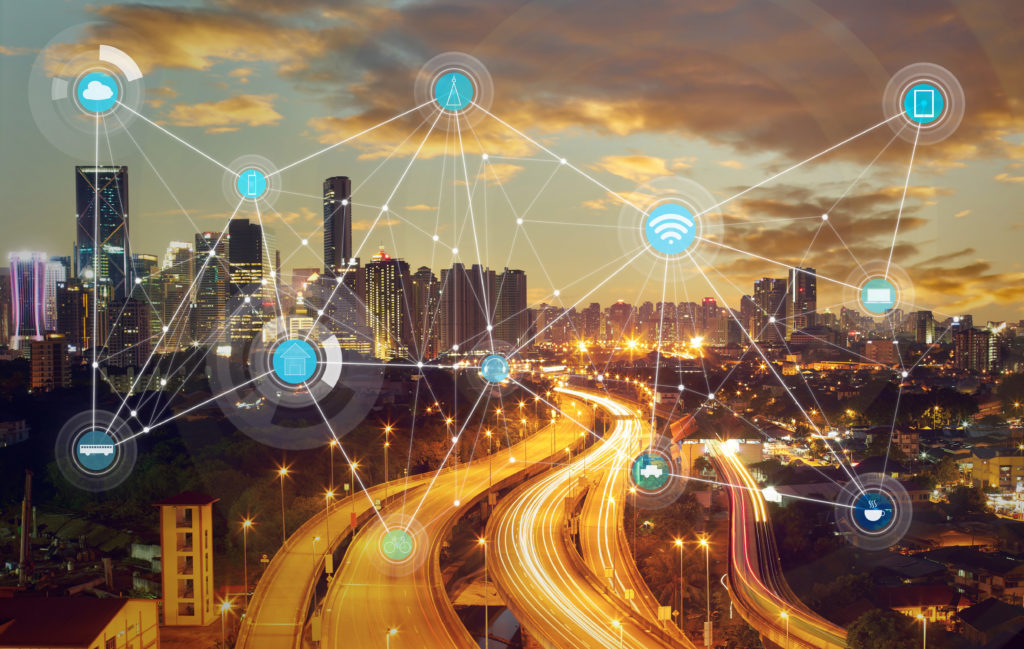
There Is a Way Out
Each year, more data is originating in the cloud, in addition to traditional on-premises data forcing companies to make a hybrid cloud shift so they can run analytics anywhere data lives. This is where SingleStore comes to the rescue.
SingleStore is a real-time data warehouse optimized for hybrid cloud deployments and excels at operational use cases. SingleStore users are drawn to the ability to load streaming data at petabyte scale while simultaneously querying that data in real-time. The distributed architecture scales for new user and data volumes with efficient, easy-to-add industry standard hardware nodes.
On-premises SingleStoreDB Self-Managed can be installed and managed by the enterprise. SingleStore supports deployment on any cloud Infrastructure as a Service, and SingleStore also has a managed service called Singlestore Helios.
There are many reasons why SingleStore can rescue you from your dated system:
- Perform faster queries with scalable high-performance SQL
- Reduced maintenance costs and hardware savings
- Run anywhere with flexible software footprint across clouds and hardware types
- Analyze all your data including stream and batch formats
- Scalable distributed architecture for growing data and users
- Enterprise-grade security for assurance across on-premises and cloud deployments
Get Started
To get started with SingleStore, please visit www.singlestore.com
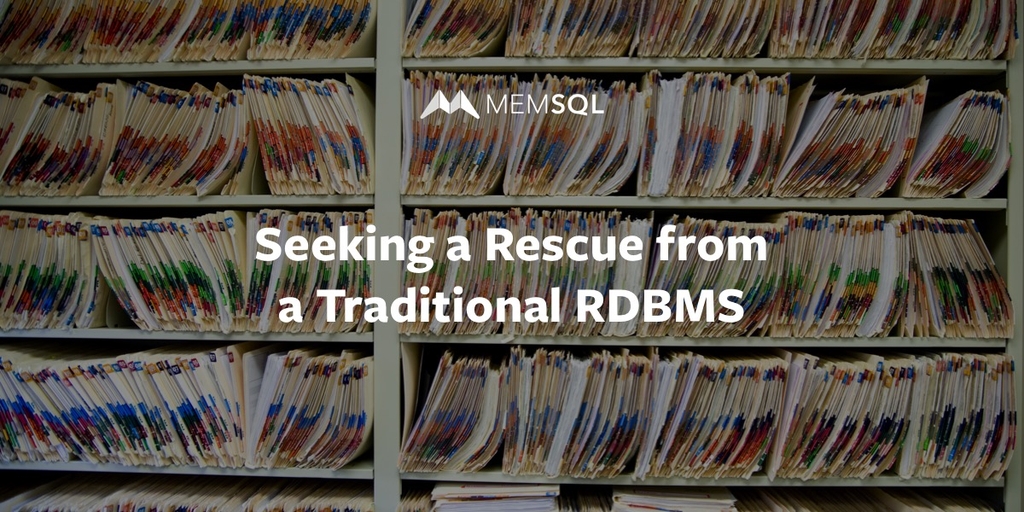