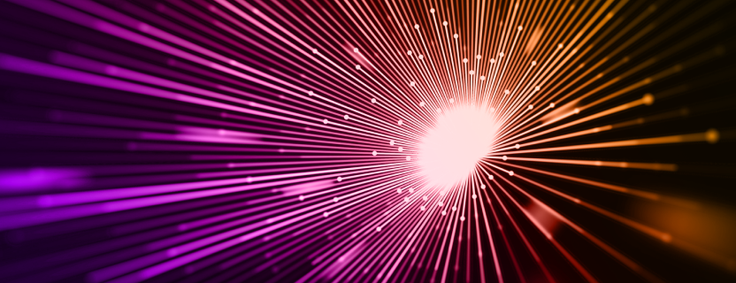
SingleStore’s unified database can set analytics and dashboards free from chronic sluggish performance. This blog is a recap of a webinar on Data Warehouse Augmentation, presented by Rick Negrin and Vijay Raja of SingleStore’s product management team. This blog captures the highlights of this webinar and includes details on three patterns that SingleStore can augment in today’s data warehouses to enable low latency, sub-millisecond performance at-scale.
Is your Data Warehouse delivering the goods?
Whether on premises or in the cloud, data warehouses have been around for decades. They’re the back-end workhorses in myriad use cases involving analytics, reporting and business intelligence (BI), querying massive amounts of aggregated data sets. But even though today’s most popular data warehouses (such as Snowflake, Amazon Redshift, Google BigQuery, Vertica and Teradata) have been born in the cloud or re-architected for it, they are increasingly challenged to meet user expectations and service level agreements (SLAs) for the real-time data ingest and millisecond query responses that are needed to power today’s data-intensive applications.
SingleStore recently put together an information-packed webinar, “20x Faster Analytics through Data Warehouse Augmentation,” that dove into the details of how our unified database can set analytics and dashboards free from chronic sluggish performance. This blog is a recap of the webinar, presented by Rick Negrin and Vijay Raja of SingleStore’s product management team, and includes details on three patterns that SingleStore can augment in today’s data warehouses to enable low latency, sub-millisecond performance at-scale.
Four types of performance bottlenecks
Let ’s jump into the webinar at about the eight-minute mark, as Vijay details the types of key challenges or bottlenecks that data warehouse workflows typically face.
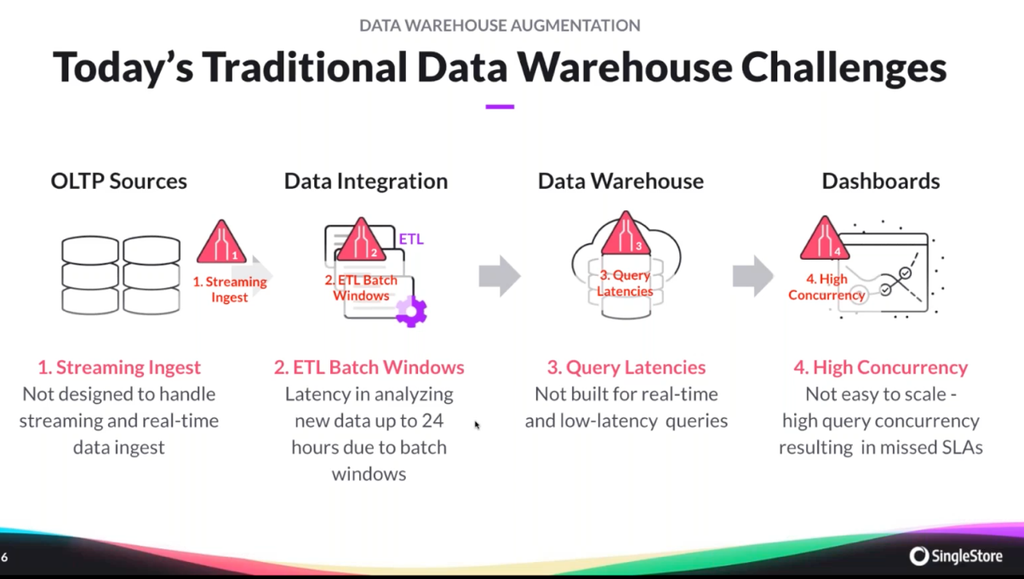
- Data warehouses are not meant, or built, to ingest and process streaming data. That’s a fundamental disconnect with what today’s users require—instant access to the freshest data in real- or near real-time.
- ETL batch windows are too long, leading to stale analytics. This impacts the downstream applications and dashboards that rely on the data, causing information or decisions to be delayed—up to six, 12 or even 24 hours depending on the batch windows.
- Query latencies are inherent, which further impede users’ ability to access and interact with the data through live dashboards or applications.
- Data warehouses are not optimized for high concurrencies, making dashboards slow to refresh. The sluggishness is particularly acute when there are hundreds of thousands of users, and/or thousands of queries running simultaneously, producing a frustrating user experience.
Key capabilities for fast analytics
To address these shortcomings, customers across diverse verticals are augmenting their data warehouses with SingleStore, powering some of their most data-intensive applications to support fast analytics. SingleStore brings three key capabilities that enable fast analytics through data warehouse augmentation:
- Ultra-fast data ingestion: SingleStore is built for parallel high throughput of up to millions of events per second, from distributed data sources such as Kafka and Spark. For example, a large bank uses SingleStore to ingest 12 million transactions per second, with over 60,000 concurrent queries per second.
- Super-low latencies: SingleStore delivers response times of 10 milliseconds or less for many customer-facing applications, with concurrency of many thousands of users and queries.
- High concurrency: SingleStore’s unparalleled scalability enables consistent performance for millions of real-time queries across tens of thousands of users—for example, a wealth management dashboard with 40,000 concurrent users.
Three ways to augment data warehouses:
Rick then took over the mic to explain ‘the how’ SingleStore augments data warehouse, describing three prominent patterns and their efficacy in specific use cases.
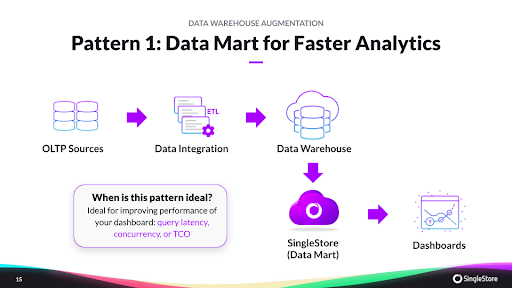
- Pattern 1: Using SingleStore as a data mart. When thousands of people are trying to simultaneously access a dashboard, the data warehouse can get overwhelmed. Query latency and concurrency become major problems. In this pattern, a subset of the information—the data needed for the dashboard—is offloaded to a SingleStore data mart. The dashboard works off this SingleStore instance, giving users the blazing fast response they demand; the data warehouse continues to handle regular reporting and other workloads. Latency drops by an order of magnitude while the dashboard serves tens of thousands of concurrent users.
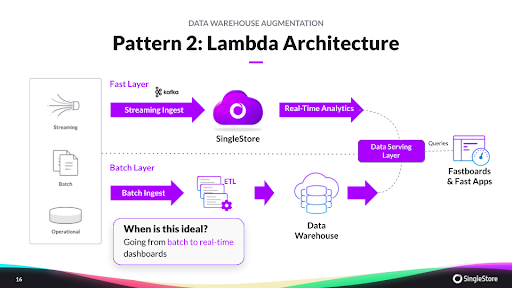
- Pattern 2: The Lambda architecture for fast analytics. This pattern is ideal when customers are leveling up from batch mode, moving to more real-time analytics. In introducing this pattern Rick said, ”From the time a piece of data is born to the time that it has to show up in the dashboard, if you had to have an SLA around that time, it would be close to or near zero.” If that’s your use case, the Lambda architecture can be an ideal choice. It’s a way of processing massive quantities of data that provides access to batch-processing and stream-processing methods with a hybrid approach.
Here, time-sensitive data or real-time data can be directly streamed into SingleStore using SingleStore Pipelines, while the rest of the data is loaded into the data warehouse via a batch-ingestion process. When queried, a serving layer merges both views to generate appropriate results.
The raw data is dropped in an event queue such as Kafka, perhaps in a single star schema with some light transformation. Aggregation is done on the fly, as part of the query to power the dashboards. SingleStore’s streaming data ingestion capabilities are a critical enabler of fast dashboard performance.
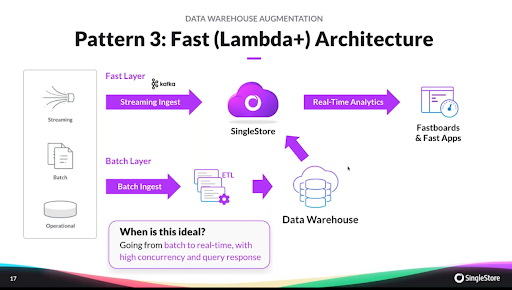
- Pattern 3: Fast or Lambda Plus architecture: Rick explained that patterns 1 and 2 are not mutually exclusive. Many SingleStore customers combine both of the patterns above because they want the benefits of the real-time and fast path patterns. Here, SingleStore performs the functions of the fast layer and the serving layer of the Lambda architecture. Customers use this pattern when they are transitioning from batch to real-time analytics ingestion, while supporting high-concurrency queries for dashboards and data-intensive applications.
Powerful use cases
The last part of the webinar was filled with detailed customer examples and Q&A with the audience. Rick walked through several impressive customer examples:
- A leading mobile phone and electronics manufacturer augmented its Teradata data warehouse with SingleStore to ingest more than four billion rows of new data daily. The response time, at a rate of 150,000 queries per second, is just 100 milliseconds!
- A leading cybersecurity and threat detection company achieved a 15x improvement in the speed of data ingestion, and a whopping 180x improvement in the time to report on the new threats, by augmenting Snowflake with SingleStore.
- A leading North American media company augmented Amazon RedShift with SingleStore and saw a 99% improvement in the speed of data ingestion, as well as a 300x improvement in latencies.
Yes, there’s more, a lot more! To hear all the technical details about the customer studies, and get a more detailed view into how SingleStore can improve the performance of data warehouse-derived analytics, watch the on-demand webinar now.
To keep up with how SingleStore is unlocking value for application developers in a wide range of industries by enabling data-intensive applications, follow us on Twitter.