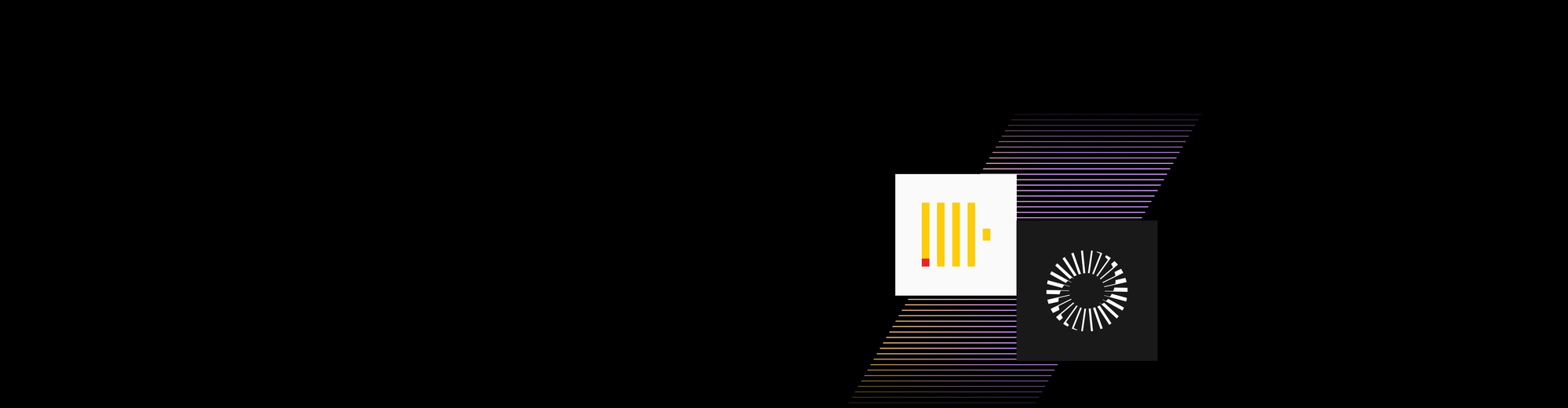
Pros and cons of real-time data warehouses
Real-time analytics stretch the limits of single-node databases, traditional data warehouses and data lakes — maxing out everything from performance to query run times, data freshness, costs and scalability.
Real-time data warehouses are built for speed, with the ability to query massive amounts of data — even at petabyte scale — within milliseconds. While ClickHouse is performant for analytical-only workloads, it falls short for speed and other data requirements beyond a single-node scope. ClickHouse also requires highly specified architecture, introducing limitations to its foundation as a real-time data warehouse.
ClickHouse works best if you:
- Work with strictly analytical data sets (no need for transactions)
Don’t need to modify data
Don’t need to worry about row retrieval
Don’t need analytics at scale (large, distributed datasets requiring joins)
Don’t need mature support for unstructured data
ClickHouse misses when you need:
- Support for unstructured and aggregated data
To find and retrieve single rows of data
A database with JSON capabilities
Support for ACID transactions
Ability to join across multiple tables
Low-latency streaming writes
Ability to stream writes to your database in real time, with sub-second and millisecond responses.
Upserts
Combination of update and insert operations, as well as using a unique key to prevent duplicate records and maintain data consistency.
Incremental deletes
Option to delete records in near real time — and sync deletes from your primary database to any analytical queries you’re running.
Comprehensive JSON support
Query, index and expand nested JSON structure, regardless of depth. And, schema flexibility to modify as needed after initial setup.
Separation of compute + storage
Better data durability, manageability, elasticity and cost advantages compared to traditional, on-premises analytical processing.
Performant joins
Enable efficient combination of large, rapidly changing data from multiple sources — without introducing significant latency.
Use cases
Running single-node workloads on Clickhouse is easy — but high-availability workloads with low-latency and high concurrency takes a database natively designed to power even your most complex apps and use cases:
Real-time analytics
Power interactive applications on data as it streams in — without losing a millisecond of query speed.
Generative AI
Leverage built-in vector search capabilities, fast K-NN, ANN vector search and full-text search.
BI and analytics dashboards
Dig deep into numbers for instant reporting, analysis and actions — and unfreeze data trapped in data lakehouses with our Iceberg integration.
Monitoring and reporting
Ingest billions of rows per second to immediately detect anomalies or fraud in cybersecurity, finance, IoT and more.
Architecture
Manage petabytes of data with a three-tier architecture comprised of memory, cache and unlimited storage.
Performance
Handle high-concurrency workloads, supporting your intelligent applications with up to hundreds of thousands of users.
Developer experience
Get up and running with a few clicks so you can quickly move to production.
Scale
Support your most complex workloads to power real-time analytics applications.
Modernizing its Teradata enterprise data warehouse to move from batch data updates to real-time streaming reports with speed and scale.
Read the case study >
Storing petabytes of data and executing distributed joins that OLAP data warehouses simply couldn’t handle. Read the case study >
Migrating to SingleStore as a full real-time data warehouse solution after struggling with Hadoop performance. Read the case study >