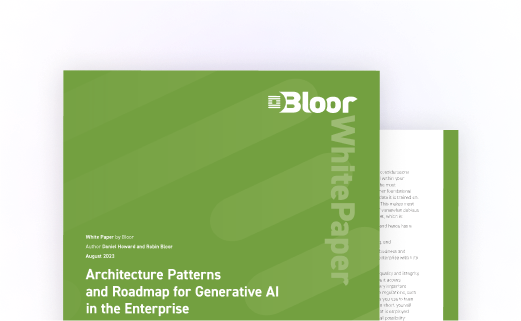
In Architecture Patterns and Roadmap for Generative AI in the Enterprise, Bloor Research lays out various options for data infrastructure available to prospective adopters of Generative AI (GAI); advises how, why, and when to build those infrastructures; and asserts that choosing and building an appropriate data infrastructure is the backbone on which GAI is built.
Key Points:
Generative AI (GAI) is the latest computing technology to take both the business world and the general public by storm. Standing on the shoulders of years of developments in data science, machine learning, and deep learning, GAI is a means to generate meaningful, easily digestible outputs from natural language prompts.
GAI engines are built from Large Language Models (LLMs), which are machine learning/predictive models driven by neural networks.
Vector database capabilities are essential to GenAI because LLMs work on the basis of vector embeddings; feeding data into an LLM requires you to represent it as a vector embedding.
Retrieval Augmented Generation (RAG) is another crucial element; RAG augments queries by feeding your LLM relevant, curated, internal enterprise data to provide additional context tailored and ensures results that are specific to your organization, data, and use case.
Key characteristics to seek when selecting a database for Generative AI include enterprise-grade functionality and scalability; the ability to combine various kinds of data, or multi-model; vector capabilities; the ability to combine transactional/operational and analytical data in a unified database; high performance; flexibility, to future-proof your GenAI deployment; and one that can readily integrate with GAI tools such as OpenAI, LangChain, and LlamaIndex.